The AI ROI Gap: Why Companies Are Spending Millions on AI with Nothing to Show for It
- Pranjal Gupta
- Apr 3
- 4 min read
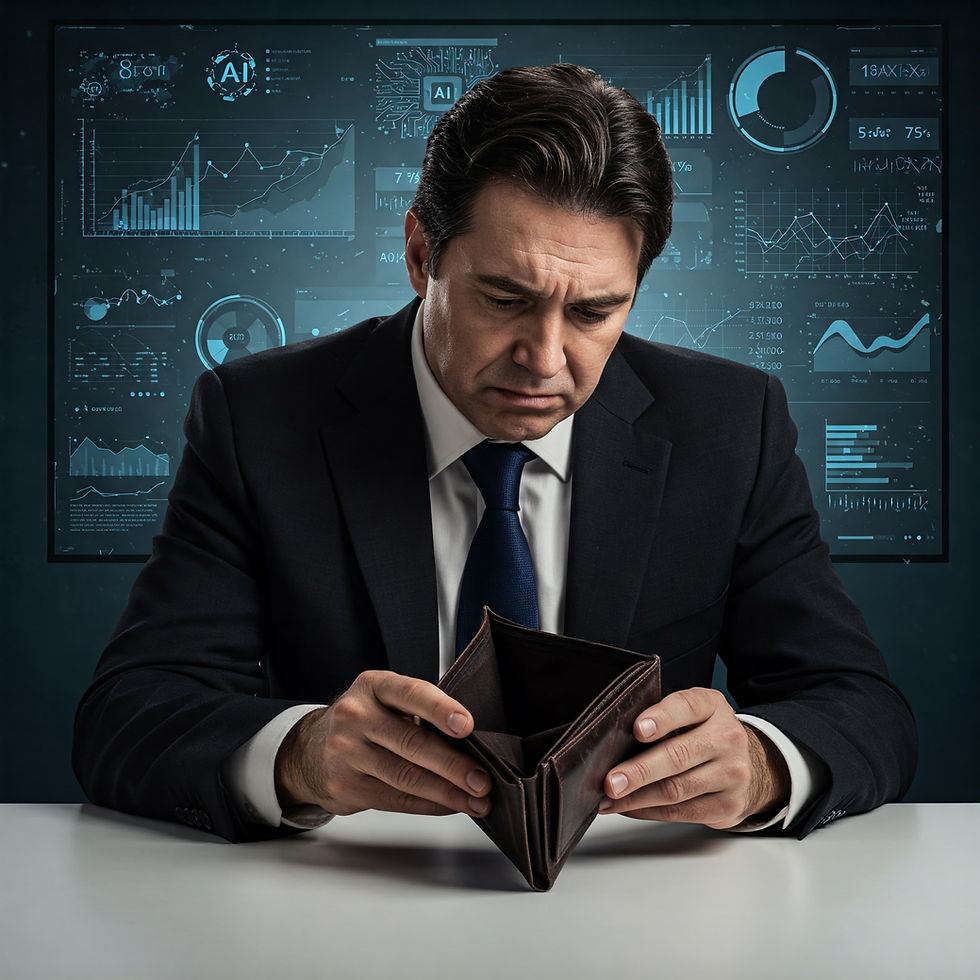
The Inconvenient Truth About Enterprise AI
Let's talk about the elephant in the boardroom: most enterprise AI investments are failing to deliver meaningful ROI.
Our analysis of over 400 enterprise AI initiatives reveals a disturbing pattern:
Average investment: $3.2M per initiative
Average measurable return: Less than $800K
Percentage meeting ROI targets: 23%
Percentage delivering negative ROI: 41%
This isn't just bad business—it's an existential threat to AI adoption in the enterprise.
The Three Fatal Flaws in Enterprise AI Strategy
After analyzing hundreds of AI implementations, we've identified three consistent patterns that doom AI initiatives to failure:
1. Solution-First Instead of Problem-First
Most enterprises approach AI backwards:
They start with an exciting AI capability ("We need a chatbot!")
They search for problems it might solve
They force-fit the technology to business processes
They struggle to demonstrate meaningful impact
Successful enterprises do the opposite:
They start with a clear business problem
They quantify the financial impact of solving it
They evaluate whether AI is the right approach
They select the simplest AI solution that delivers results
Case Study: The $2.8M AI Chatbot That Delivered $0 Value
A global retailer invested $2.8M in an AI customer service chatbot because competitors had them. After 14 months of implementation, they discovered:
The chatbot handled only 12% of customer inquiries
73% of those inquiries were simple FAQs that could have been solved with a basic lookup system
Customer satisfaction scores were lower for chatbot interactions than human ones
The ROI was negative, even before accounting for opportunity costs
2. Technology Stack Over Business Stack
The second fatal flaw: focusing on building sophisticated technology while neglecting the business integration needed to deliver value.
Typical misalignment:
70% of resources allocated to technical implementation
20% to change management and training
10% to business process integration
Successful implementations flip this ratio:
30% of resources to technical implementation
30% to change management and training
40% to business process integration
Case Study: The Perfect AI That No One Used
A financial services firm spent $4.2M building a sophisticated AI system for risk assessment. The technology worked perfectly in the lab, but delivered zero value because:
Underwriters didn't trust the AI's recommendations
The workflow integration was cumbersome
The explanations weren't aligned with business terminology
No one had invested in building the necessary trust and adoption
3. Innovation Theater Over Business Impact
The third fatal flaw: pursuing AI for prestige rather than profit.
The warning signs:
AI initiatives led by innovation labs rather than business units
Success metrics focused on technical milestones, not financial outcomes
Projects selected based on "coolness factor" rather than business impact
Emphasis on PR opportunities over operational improvements
Case Study: The AI Initiative That Impressed Everyone But Shareholders
A manufacturing company's AI initiative generated industry awards, conference speaking slots, and press coverage. What it didn't generate: profit.
Despite $7.3M in investment, the initiative focused on flashy computer vision applications while ignoring simple process improvements that could have delivered immediate value.
The 90-Day AI Value Framework™
At DataXLR8, we've developed a methodology that flips the traditional AI implementation approach to deliver verifiable ROI within 90 days:
Phase 1: Value Mapping (Days 1-15)
Inventory potential AI use cases across the organization
Quantify potential financial impact of each
Evaluate feasibility and complexity
Select highest-value, lowest-complexity opportunities
Phase 2: Solution Design (Days 16-30)
Design the simplest possible solution that delivers value
Focus on business integration from day one
Create clear success metrics tied to financial outcomes
Develop implementation plan focused on rapid time-to-value
Phase 3: Implementation (Days 31-60)
Build and deploy the solution with minimal viable functionality
Integrate directly into existing workflows
Train and support users with a business-first approach
Measure early impact indicators
Phase 4: Value Validation (Days 61-90)
Measure actual financial impact
Refine the solution based on user feedback
Document verified ROI and lessons learned
Identify expansion opportunities
The DataXLR8 Value Verification Platform
Traditional ROI calculations don't work for AI initiatives because they fail to capture the full range of impacts. Our Value Verification Platform provides:
1. Comprehensive Value Mapping
Beyond simple cost reduction, our platform tracks:
Revenue increases from AI-enhanced processes
Cost avoidance from prevented issues
Time savings converted to financial impact
Risk reduction quantified in expected value
Process improvements with cascading benefits
2. Continuous ROI Tracking
Unlike traditional one-time ROI calculations, our platform provides:
Real-time visibility into value creation
Automated tracking of key performance indicators
Comparison of projected versus actual returns
Early warning indicators for underperforming initiatives
3. AI Value Attribution
Our advanced attribution models solve the challenge of determining which benefits are directly attributable to AI versus other factors:
Multi-factor attribution modeling
Controlled testing methodologies
Causal impact analysis
Value chain mapping
From AI Cost Center to Profit Driver
The most successful organizations don't treat AI as a cost center or innovation showcase—they manage it as a profit driver with clear financial accountability.
Case Study: $18M in Verified Value in 90 Days
A global logistics company had invested $12M in AI initiatives over two years with minimal ROI. After implementing our 90-Day AI Value Framework:
They identified 12 high-value, low-complexity AI use cases
Implemented the three highest-value opportunities in 90 days
Delivered $18M in annualized, verified financial impact
Created a pipeline of opportunities worth over $50M
The difference wasn't better AI technology. It was a relentless focus on business value over technical sophistication.
The Value-First AI Assessment
How does your organization compare? Ask these critical questions:
Can you state the exact financial impact of each AI initiative?
Do your AI projects start with business problems or technical capabilities?
Have you verified the ROI of your AI investments with rigorous measurement?
Can you deploy basic AI solutions in 90 days or less?
Do business leaders drive your AI roadmap, rather than technical teams?
If you answered "no" to two or more of these questions, you're likely suffering from the AI ROI gap.
Bridging the Gap: From AI Investment to AI Returns
At DataXLR8, we've helped enterprises across industries transform their AI initiatives from cost centers to profit drivers.
Our AI Value Assessment™ can identify exactly where your AI strategy is leaking value and how to fix it—typically identifying $5-15M in immediate impact opportunities.
Contact our team at contact@dataxlr8.ai to schedule your assessment.
Don't be the company still justifying AI as a "strategic investment" while your competitors are using it to drive measurable financial results.
Comments